The goal is to make 6G systems the infrastructure of a new generation of mobile, intelligent, and context-sensitive services that are available everywhere with high trustworthiness and performance and can draw on both network-side (e.g., JC&S) and network-external context sources such as sensors. In addition, the networks themselves should become smarter and thus more efficient and resource-efficient, which requires a high degree of automated use of AI. Consideration is also given to the additional resources required in the network to efficiently execute network-side or third-party services (optimized HW resources for AI, computing resources for communication-control codesign, …). Methodological aspects such as modeling and data synthesis are also considered.
The goals of the AP are to develop an overall concept for networking, processing and providing location/sensing and other network-side context information in 6G with open APIs for third parties, to develop an overall concept for optimized provisioning/instantiation of AI functions (sensor, data filtering and fusion, rating, learning, interference, … ) for internal and external services taking into account specific HW resources in the network, network properties and resource and energy efficiency and performance, integration of functionalities to support special requirements for data access rights in AI based multi-party ecosystems (federated learning, transfer learning, …) to rights to data of open ecosystems with independent parties and the development of a concept to support provability and trustworthiness of information and context sources (distributed ledger technologies in the network, trust modules as resource, …).
Project goals
- Networking of functionalities
- Making data available
- Modeling and data synthesis with data from all linked work packages
- Context management in 6G networks
- Provability and trustworthiness of data and context sources with trust anchors and blockchain
- Flexibility & extensibility of 6G campus networks
- Performance monitoring of in-network context management
- AI Management
- Management of AI functions
- Resource-dependent optimization
- Distributed AI
- Multi-party AI concepts incl. rights management
- Federated Learning, Transfer Learning
Role in project
- Definition of procedures and algorithms that enable further processing by AI procedures.
- Central interface to relate the data of RAN, core and security/resilience to each other.
- Integrated into the overall system through the architecture and also used in a special form for campus networks
Results and achievements
- Development of a general service-oriented communication platform including context management system
- Design of an abstraction layer to use said communication platform for building organic infrastructures
Resources
- NVIDIA DGX A100
- ML/KI High Performance Data Center
Contacts
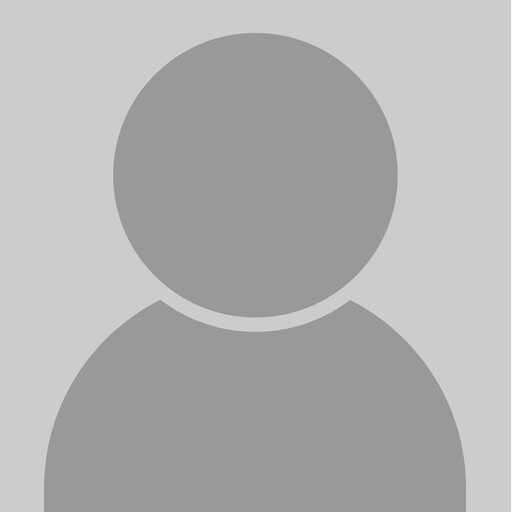
Dennis Krummacker
Head
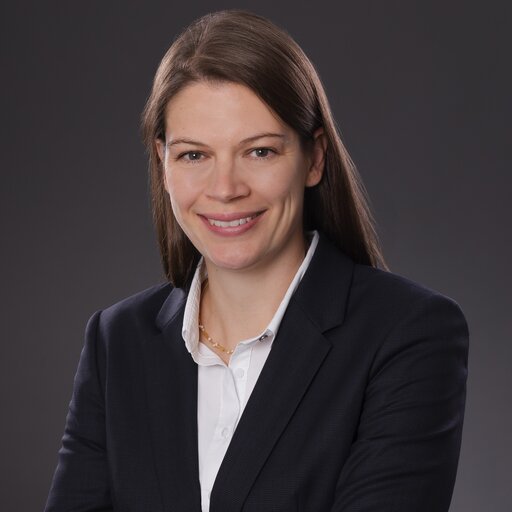
Anke Schmeink
Deputy Head
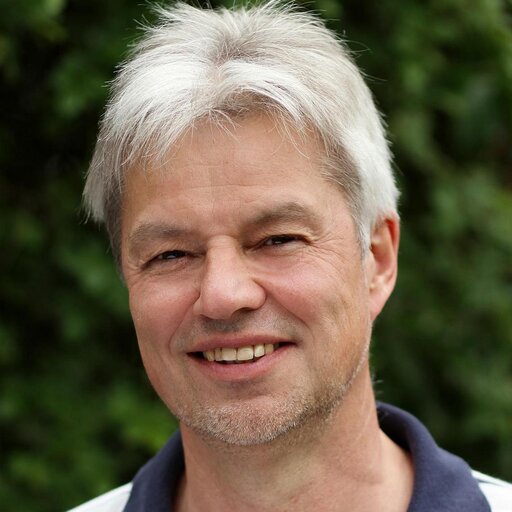
Prof. Norbert Wehn
Domain Expert